Ir.ia.ac.cn
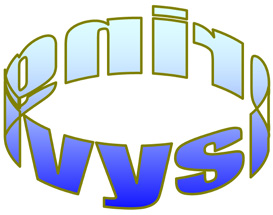
Int. J. Med. Sci. 2016, Vol. 13
International Publisher
International Journal of Medical Sciences
2016; 13(2): 99-107. doi: 10.7150/ijms.13456
Comorbidity Analysis According to Sex and Age in
Hypertension Patients in China Jiaqi Liu1†, James Ma3†, Jiaojiao Wang1†, Daniel Dajun Zeng1, Hongbin Song4, Ligui Wang4, Zhidong
1. The State Key Laboratory of Management and Control for Complex Systems, Institute of Automation, Chinese Academy of Sciences, Beijing, China;
2. Cloud Computing Center, Chinese Academy of Sciences, Dongguan, China.
3. College of Business, University of Colorado, Colorado Springs, CO, USA.
4. Institute of Disease Control and Prevention, Academy of Military Medical Sciences, Beijing, China.
†These authors contributed equally to this work.
Corresponding author: Zhidong Cao, Institute of Automation, Chinese Academy of Sciences, No 95 Zhongguancun East Road, 100190, Beijing, China. E-mail:
[email protected]
Ivyspring International Publisher. Reproduction is permitted for personal, noncommercial use, provided that the article is in whole, unmodified, and properly cited. See
http://ivyspring.com/terms for terms and conditions.
Received: 2015.08.04; Accepted: 2015.11.11; Published: 2016.01.29
Abstract
Background: Hypertension, an important risk factor for the health of human being, is often accom-
panied by various comorbidities. However, the incidence patterns of those comorbidities have not been
widely studied.
Aim: Applying big-data techniques on a large collection of electronic medical records, we investigated
sex-specific and age-specific detection rates of some important comorbidities of hypertension, and
sketched their relationships to reveal the risk for hypertension patients.
Methods: We collected a total of 6,371,963 hypertension-related medical records from 106 hospitals
in 72 cities throughout China. Those records were reported to a National Center for Disease Control
in China between 2011 and 2013. Based on the comprehensive and geographically distributed data set,
we identified the top 20 comorbidities of hypertension, and disclosed the sex-specific and age-specific
patterns of those comorbidities. A comorbidities network was constructed based on the frequency of
co-occurrence relationships among those comorbidities.
Results: The top four comorbidities of hypertension were coronary heart disease, diabetes, hyper-
lipemia, and arteriosclerosis, whose detection rates were 21.71% (21.49% for men vs 21.95% for
women), 16.00% (16.24% vs 15.74%), 13.81% (13.86% vs 13.76%), and 12.66% (12.25% vs 13.08%),
respectively. The age-specific detection rates of comorbidities showed five unique patterns and also
indicated that nephropathy, uremia, and anemia were significant risks for patients under 39 years of age.
On the other hand, coronary heart disease, diabetes, arteriosclerosis, hyperlipemia, and cerebral in-
farction were more likely to occur in older patients. The comorbidity network that we constructed
indicated that the top 20 comorbidities of hypertension had strong co-occurrence correlations.
Conclusions: Hypertension patients can be aware of their risks of comorbidities based on our
sex-specific results, age-specific patterns, and the comorbidity network. Our findings provide useful
insights into the comorbidity prevention, risk assessment, and early warning for hypertension patients.
Key words: Hypertension, Comorbidity, Electronic Medical Records, Detection Rate, Network Analysis.
Background
Hypertension, or high blood pressure, is one of
2]. In China, hypertension is the leading preventable
the most important risk factors that can lead to car-
risk factor for death among Chinese adults aged 40
diovascular diseases, and is thus regarded as a serious
years and older [3, 4]. Moreover, hypertension has a
public health problem. The prevalence of hyperten-
large number of comorbidities, which greatly affect
sion has been increasing in most areas worldwide [1,
hypertension patients' quality of life [5-7]. In previous
http://www.medsci.org
Int. J. Med. Sci. 2016, Vol. 13
years, researchers and medical practitioners have
Although the comorbidities of hypertension
made a tremendous effort to study the comorbidities
have been extensively studied, most existing research
of hypertension [8-10]. Specifically, heart disease [2,
is based on medical surveys and public census data.
11], diabetes [12, 13], and obesity [14, 15] are the most
Census data sets show aggregated facts of the general
widely studied comorbidities of hypertension. Some public without detailed information regarding indi-
other diseases, such as allergic respiratory disease [9],
vidual patients. In contrast, medical surveys while
sleep-disordered breathing [16], and chronic kidney include some individual level information usually
disease [17], have also been studied as potential involve a limited number of survey participants be-
comorbidities of hypertension. Hypertension and cause of limited resources. Those surveys are often set
some of its comorbidities have shown high correla-
for a confined geographical area (i.e., a city or a
tions in terms of their prevalence. An example of this
county), and thus cannot claim to be representative of
type of correlations is that the prevalence of hyper-
a larger and broader area. Due to the nature of medi-
tension in patients with diabetes is as high as 92.7% cal surveys, usually only certain types of participants
are willing to reveal their private and sensitive medi-
Moreover, the sex-specific and age-specific
cal related situations. People with a stronger sense of
analyses of comorbidities of hypertension have re-
privacy are normally reluctant to reveal their medical
sulted in various important findings [1, 19-21]. Spe-
history or health-related conditions. Therefore, med-
cifically, the incident rates of comorbidities in hyper-
ical surveys on a voluntary basis may have a biased
tension patients with a different sex and age can sig-
participant population. The data points that are col-
nificantly differ. An example of this difference is that
lected in a medical survey also largely depend on the
the incidence of hypertension and hypercholesterol-
participant's availability during the time of the sur-
emia combined is 20% for women versus 16% for vey, the participant's mood at the time, and the sur-
men, and ranges from 1.9% for those aged 20–29 to vey collector's attitude and human interaction skills.
56% for those aged 80 years and older [22]. Addition-
Too many human-related factors can affect the quality
ally, patient's age and sex need to be considered for of medical surveys. Furthermore, to reduce the survey
treatment of these comorbidities [23, 24]. An example
participant's reluctance, a medical survey is usually
of the situation is that treatment for hypertension pa-
composed of a limited number of survey questions so
tients who are 80 years or older with indapamide has
that an interview or a questionnaire can be completed
been proved to be effective and can also reduce the within a short time period. This greatly reduces the
patient's risk of stroke [23]. Research has shown that
versatility of the survey when analyzing the survey
untreated male hypertension patients are more likely
results. In summary, because of the time-consuming
to suffer from cognitive impairment than untreated and labor-intensive nature of medical surveys, the
female hypertension patients do [25]. Thus, hyper-
limited number of, and possibly biased, survey par-
tension should be treated and controlled as early as ticipants and survey questions can lead to biased
possible for male patients before they encounter de-
analysis results, and possibly overlook important
patterns and relationships in the occurrence of dis-
There has been increasing interest in analyzing eases.
disease relationships using network theory [26, 27].
In the current study, we leveraged a large, relia-
The disease network is particularly useful when ana-
ble, and extensive data set and analyzed the occur-
lyzing the co-occurrence of different diseases. Specif-
rence patterns of hypertension comorbidities. We also
ically, the disease network denotes an individual investigated the common comorbidities of hyperten-
disease with a vertex, and the co-occurrence of two sion with respect to the patient's sex and age. The
diseases with an edge connecting those two diseases.
co-occurrence relationships among comorbidities of
The disease network summarizes the connections hypertension are also discussed using the disease
among diseases and shows progress of disease pref-
network approach.
erentially along the edges or links [28]. The frequency
of co-occurrence relationships among important Methods
comorbidities could provide useful insight into de-
scribing the disease development process, and thus Study population
result in doctor's and patient's awareness of diseases
Our data set was obtained from a Chinese Na-
at the early stage of development. Studying the tional Surveillance System, which was initially im-
comorbidity co-occurrence of hypertension using the
plemented by the Chinese government in 2010. This
disease network may be an effective tool for deter-
surveillance system collects electronic medical records
mining meaningful comorbidity relationships that from hospitals and aims to oversee the overall health
other approaches have not reported.
conditions of the Chinese population. Since 2010, this
http://www.medsci.org
Int. J. Med. Sci. 2016, Vol. 13
system has been adopted by 192 hospitals located
Statistical analysis
throughout China. Although we had access to all 192
The occurrences of comorbidities were counted
hospitals' data in the surveillance system, we inten-
in hypertension-related electronic medical records.
tionally excluded some hospitals that did not appear
The comorbidity's occurrence was then utilized to
to present a sufficient and continuous data stream. derive the detection rate of the comorbidity which
Some obvious errors and incomplete data points were
better reflected the comorbidity's prevalence in hy-
also removed to maintain the data integrity.
pertensive patients. The detection rate of a comorbid-
Eventually, we decided to use 6,371,963 hyper-
ity was defined as the ratio of the number of the
tension-related high-quality data records from the comorbidity's records to the number of hyperten-
110,528,991 electronic medical records that we had sion-related records:
access to. Those medical records were dated between
2011 and 2013, and were from 106 hospitals located in
72 cities in China (Figure S1). Those cities are geo-
graphically distributed in 29 of 31 provinces in China
The sex-specific detection rate was determined
(excluding two underpopulated provinces, Qinghai as the ratio of the number of each comorbidity in
and Ningxia). Our data set covers 33.90% of the city
males or females to the number of hypertension cases
population in China. The city population data is based
in the corresponding sex group. The odds ratios and
on the sixth Chinese population census published by
their 95% confidence interval (CI) of each sex-specific
the National Bureau of Statistics of the People's Re-
detection rate were also calculated. For the
public of China (http://www.stats.gov.cn).
age-specific analysis, every 10-year age range between
This study was approved by the institutional re-
0 and 99 years was considered an age group (e.g., 0–9
view board of the Institute of Automation, Chinese years, 10–19 years). Ages greater than or equal to 100
Academy of Sciences. The data set was collected by years were considered as one age group. Because the
the Chinese government for disease control. All pa-
numbers of each comorbidity in the 0–9 years group
tients gave their informed consent. The patient's pri-
and above 99 years group were small, the age-specific
vacy was strictly preserved in our study. We only detection rates were calculated and analyzed only
used the patient's sex, age, and clinical diagnostic from 10 years to 99 years. Similarly the age-specific
information to perform our analysis. Patients' identi-
detection rate was determined as the ratio of the
ty-related information was masked before we started
number of each comorbidity in each age group to the
number of hypertension cases in the corresponding
Data normalization
age group. Their 95% CIs were calculated. To analyze
The clinical diagnosis in the original electronic the age-specific prevalence trends of the top 20
medical records was not coded using uniformed and
comorbidities, the expectation maximization class in
standardized text terms. An example was that some Weka [32] version 3.7.7 was used to cluster those 20
doctors had used "upper infection" as an abbreviation
trends. The expectation maximization [33] algorithm
for "upper respiratory tract infection" and others had
assigns a probability distribution to each trend, which
chosen a different abbreviation for the same diagno-
indicates the probability of it belonging to each clus-
sis. To standardize the diagnosis, we applied a natural
language processing technique [29, 30] and developed
Network analysis
several in-house Python scripts for Chinese text pro-
When two comorbidities of hypertension ap-
cessing and mining. Python [31] has been proved an
peared in one electronic medical record, we consid-
effective tool for handling similar tasks. Specifically ered that there was a co-occurrence relationship be-
for our study, each electronic medical record was au-
tween this comorbidity pair. The number of
tomatically segmented into a series of Chinese words,
co-occurrences between a couple comorbidities can be
and these words were then combined to form Chinese
an important factor to reveal the relationship of those
phrases according to the probability distribution of two comorbidities. Thus, we constructed a weighted
those words. In addition to automatic normalization comorbidity network [34, 35] to study the comorbidi-
of data, many text ambiguities and synonyms were ties of hypertension and the co-occurrence relation-
handled manually. Finally, all medical diagnostic ships among those comorbidities.
records were converted to standardized and coded
The nodes of the network represented comor-
diagnostic terms that could be easily manipulated and
bidities and the diameter of each node was propor-
tional to the detection rate of each comorbidity. An
edge in the network indicated the co-occurrence of
two comorbidities whom that edge was connecting.
http://www.medsci.org
Int. J. Med. Sci. 2016, Vol. 13
The weight of an edge was the number of sufficiency and uremia, and respiratory-related dis-
co-occurrences of those two comorbidities. When an eases, such as respiratory tract infection, upper res-
electronic medical record contained more than two piratory tract infection, and tracheitis had a high de-
comorbidities of hypertension, the count of every re-
tection rate, which indicated that those comorbidities
lationship between each possible pair of comorbidities
were of a higher risk in hypertension patients than
in that record would have an increment of one (e.g., other comorbidities were. Moreover, the detection
when the record was "hypertension, A, B, C", the rates of comorbidities reduced with rank. The detec-
count of relationships A-B, A-C, and B-C would all
tion rate of the last comorbidity, arthritis, was only
encounter an increment of one). After investigating all
hypertension-related electronic medical records, we
retained the high-frequency relationships among the
top 20 comorbidities. The high-frequency relation-
Table 1. Detection rates of the top 20 comorbidities of hyper-
ships were defined as relationships with a weight of tension in China.
more than 1% of the total number of hyperten-
sion-related records.
Coronary Heart Disease
Several network measures have been adopted to
identify the importance of nodes [36]. Three primary
methods, namely degree centrality, average degree, 4
Arteriosclerosis
and average path length [37], were used to analyze the
Cerebral Infarction
Move With Difficulty
comorbidity network. Degree centrality is the most 7
readily calculated and understood concept of node 8
Respiratory Tract Infection
centrality. The degree centrality of a comorbidity is 9
Cerebral Circulation Insufficiency 3.87
the total number of relationships that are directly as-
Upper Respiratory Tract Infection 3.43
Renal Insufficiency
sociated with that comorbidity. A comorbidity with a
high degree centrality has more co-occurrence rela-
tionships with other comorbidities in the network 14
[38]. The average degree of a network is an overall 16 Anemia
evaluation about the connections among comorbidi-
ties [39]. In addition, path length focuses on the least
number of relationships in order to connect two 19
Osteoarthropathy
comorbidities. A comorbidity pair with a low path
length and high edge weights along the path has a
higher risk of co-occurrence in hypertension patients.
The path length of any two directly connected Sex-specific detection rates
comorbidities is one and the number of comorbidities
The sex-specific detection rates of the top 20
on the shortest path is path length minus one. Similar
comorbidities of hypertension and their odds ratios
to the average degree of a network, the average path
were shown in Table 2 and Figure S2. Osteoporosis
length of a network is also used to describe the aver-
showed the largest difference between males and fe-
age distance between each comorbidity pair in the males, which suggested that female hypertension pa-
network [40]. A frequently used force-directed layout
tients have a 73.12% higher risk than male hyperten-
algorithm, the Fruchterman–Reingold algorithm, was
sion patients in developing osteoporosis. Other
used to layout the network.
bone-related diseases, such as arthritis and osteoar-
thropathy, also had a higher incidence in female hy-
pertension patients than in male hypertension pa-
Detection rates of the top 20 comorbidities
tients (40.64% vs 36.29%). In addition, insomnia and
The top 20 comorbidities of hypertension with
difficulty with movement threated the health of fe-
the highest detection rates were identified (Table 1). males more than males (39.78% vs 29.92%). Surpris-
Coronary heart disease (CHD), which is one of the ingly, two cerebral diseases showed different risks in
most important cardiovascular diseases, had the males and females. Cerebral circulation insufficiency
highest detection rate. Diabetes, hyperlipemia, and was 40.15% more likely to occur in females, while
arteriosclerosis had a detection rate that was higher cerebral infarction was 19.05% more likely to occur in
than 10%. Cerebral diseases, such as cerebral infarc-
males. Moreover, several diseases related to the kid-
tion and cerebral circulation insufficiency, kid-
ney had a higher morbidity in male hypertension pa-
ney-related diseases, such as nephropathy, renal in-
tients than in female hypertension patients. More at-
tention should be paid to renal insufficiency, uremia,
http://www.medsci.org
Int. J. Med. Sci. 2016, Vol. 13
and nephropathy in male hypertension patients pertension patients was cerebral infarction being
(35.39%, 25.56%, and 17.99%) than in female hyper-
ranked in the top five comorbidities between 50 and
tension patients. The sex-specific detection rates of 89 years of age and the fourth at 90–99 years of age.
other top comorbidities, including CHD, diabetes,
The age-specific detection rates of the top 20
hyperlipemia, and arteriosclerosis, were relatively comorbidities of hypertension (Figure 2) were clus-
uniform, with no significant differences between male
tered into five classes. First, the age-specific detection
and female patients.
rates of CHD, arteriosclerosis, cerebral infarction, in-
somnia, arrhythmia, gastritis, osteoarthropathy, and
Age-specific detection rates
arthritis gradually increased as patients got older. The
The age-specific occurrence distribution of hy-
detection rates of these comorbidities at 90–99 years
pertension patients was shown in Figure 1. Based on
were several times (relative ratio: CHD, 25.91; arteri-
6,371,963 electronic medical records, the proportion of
osclerosis, 22.65; cerebral infarction, 18.55; insomnia,
hypertension patients who were aged between 50 and
12.58; arrhythmia, 8.17; gastritis, 3.03; osteoarthropa-
79 years was 71.27% (95% CI: 71.23–71.31%). Only thy, 62.16; and arthritis, 8.02) higher than those at the
5.99% of hypertension patients were younger than 40
age of 10–20 years.
years. In addition, because there was only a small
number of patients who were aged 9 years or older
than 100 years, these two age groups were removed
from the analysis.
The top five detection rates of comorbidities in
each age group were different (Table 3). Nephropa-
thy, uremia, and anemia were the three biggest risks
for hypertension patients who were younger than 39
years, while renal insufficiency was a potential risk to
hypertension patients who were younger than 29
years. Hyperlipemia was always in the top five
comorbidities through all age groups and was the top
comorbidity in the 40–49-year age group. Addition-
ally, CHD, diabetes, and arteriosclerosis became a
major risk when hypertension patients were older
than 40 years. Another significant risk for older hy-
Figure 1. Age-specific distribution of hypertension patients in China.
Table 2. Sex-specific distribution of the top 20 comorbidities of hypertension in China.
Male Detection Rate(%) 95% CI
Female Detection Rate(%) 95% CI
Odds ratios 95% CI
Coronary Heart Disease
21.44-21.53 21.95
21.90-22.00 0.973
0.970-0.977 <.00001
16.20-16.28 15.74
15.70-15.78 1.038
1.034-1.042 <.00001
13.82-13.89 13.76
13.72-13.80 1.008
1.003-1.012 0.00057
Arteriosclerosis
12.22-12.29 13.08
13.05-13.12 0.928
0.923-0.932 <.00001
Cerebral Infarction
1.200-1.215 <.00001
Move With Difficulty
0.761-0.773 <.00001
1.179-1.198 <.00001
Respiratory Tract Infection
0.892-0.907 <.00001
Cerebral Circulation Insufficiency 3.24
0.698-0.710 <.00001
Upper Respiratory Tract Infection 3.36
0.945-0.962 <.00001
Renal Insufficiency
1.355-1.380 <.00001
0.946-0.963 <.00001
0.563-0.573 <.00001
0.702-0.715 <.00001
1.252-1.276 <.00001
0.969-0.988 2.5E-05
0.908-0.927 <.00001
0.868-0.886 <.00001
Osteoarthropathy
0.721-0.737 <.00001
0.698-0.714 <.00001
http://www.medsci.org
Int. J. Med. Sci. 2016, Vol. 13
Table 3. Top five comorbidities of hypertension in each age group.
Renal Insufficiency
Renal Insufficiency
Arteriosclerosis
Arteriosclerosis
Cerebral Infarction
Arteriosclerosis
Cerebral Infarction
Arteriosclerosis
Cerebral Infarction
Arteriosclerosis
Cerebral Infarction
Arteriosclerosis
Cerebral Infarction
Figure 2. Age-specific patterns of the top 20 comorbidities of hypertension in China.
Second, the age-specific detection rate of diabe-
Third, moving with difficulty, respiratory tract
tes, hyperlipemia, and cerebral circulation insuffi-
infection, upper respiratory tract infection, tracheitis,
ciency increased with an increase in age but decreased
and osteoporosis had different rates of detection rate
in older patients. For diabetes and hyperlipemia, the
increase, and occasionally showed a slight decline
detection rate reached a peak at 70–79 years, with depending on age. The detection rate of moving with
detection rates of 19.55% and 15.33%, respectively. difficulty greatly increased at 20–29 years (228.86%),
The detection rate of diabetes continued to increase
50–59 years (172.18%), and 70–79 years (153.28%)
over time, with the highest detection at 40–49 years. compared with the previous age group. Respiratory
However, the detection rate of hyperlipemia flattened
tract infection and upper respiratory tract infection
off from 50–79 years (14.56–15.33%). Moreover, the had the same patterns in detection rate of greatly in-
detection rate of cerebral circulation insufficiency creasing at 20–29 years (171.34% and 153.13%, respec-
flattened off from 50–69 years (3.92%) and then tively) and 50–59 years (128.87% and 127.20%, respec-
peaked at 70–89 years (4.62–4.70% for the two age tively). The detection rate of tracheitis greatly in-
groups). The detection rates of these three comorbidi-
creased at almost all age ranges (131.13–181.66%) and
ties greatly decreased in older people after the peak declined at 60–69 years (103.18%) compared with
(diabetes: 29.72%; hyperlipemia: 26.01%; and cerebral
previous age groups. The detection rate of osteoporo-
circulation insufficiency: 8.05%).
http://www.medsci.org
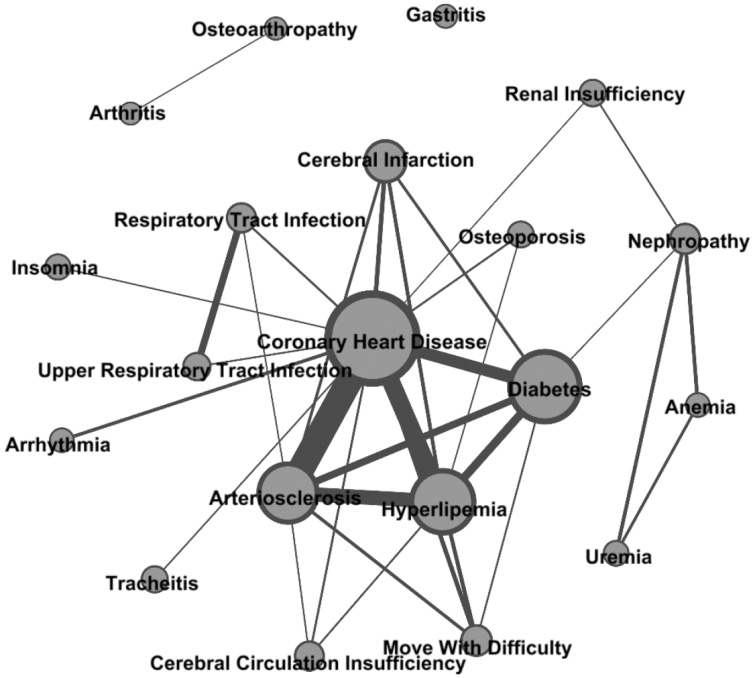
Int. J. Med. Sci. 2016, Vol. 13
sis was also unique in that it decreased below 40 tion ensured individual patient's medical records to
years and quickly increased by 50–59 years (212.36%).
be reliable, extensive, and timely. Compared with
Fourth, the trend for detection rate did not al-
other similar research [1, 19, 20, 22], our study was
ways show an upward trend. The detection rate of based on a much larger patient base. Our data records
kidney-related diseases continued to fall at most age
were collected while patients were hospitalized, thus
ranges. The age-specific detection rates of nephropa-
the records contained detailed and extensive coverage
thy, uremia, and anemia fell from 16.81% (10–19
on patient's medical-related information. Because the
years) to 1.94% (90–99 years), 10.47% (20–29 years) to
medical-related information was for medical diag-
0.56% (90–99 years), and 8.57% (10–19 years) to 1.64%
nostic purposes, the information was highly reliable
(80–89 years), respectively. The decline in detection and objective.
rate of these diseases in each age group compared
with the previous age group was similar.
The last class only contained renal insufficiency
whose detection rate reached a peak at 20–29 years
(6.15%) and showed a U-shaped curve with increasing
age. At 50–69 years old, hypertension patients had the
lowest risk in developing renal insufficiency with a
detection rate of only 2.66%.
Comorbidity network of hypertension
The comorbidity network comprising high
co-occurrence frequency relationships among comor-
bidities of hypertension was presented in Figure 3 and
Table S1. The core of the network included CHD, hy-
perlipemia, arteriosclerosis, and diabetes whose de-
gree centrality was 13, 7, 7, and 6, respectively. Those
comorbidities were directly connected to 75% of all
comorbidities. Therefore, hypertension patients who
had one of those four comorbidities had a greater
health risk. Uremia and anemia were connected to the
Figure 3. Comorbidity network of hypertension.
core network through nephropathy, which indicated
that nephropathy was an important indicative varia-
ble between those two comorbidities and the core
To the best of our knowledge, this study was the
network. Hypertension patients with CHD, hyper-
first to investigate the prevalence of comorbidities of
lipemia, arteriosclerosis, and diabetes had a relatively
hypertension through a large amount of electronic
low risk of developing uremia and anemia. Gastritis medical record data rather than using just medical
and the comorbidity pair of arthritis and osteoar-
survey or census data. Our findings on the detection
thropathy were isolated in the core network. The rates of comorbidities were sufficiently representative
morbidity risk of these three comorbidities was rela-
for Chinese population and were insightful for doc-
tively independent to comorbidities in the core net-
tors and hypertension patients. The top 20 comorbid-
work. Moreover, because the average degree was 3.3
ities in terms of the detection rate and their
and the average path length was 2.09 in the comor-
co-occurrence relationships implied important health
bidities network, the comorbidity network showed risks to hypertension patients. From our study, more
that the top 20 comorbidities had a strong correlation
targeted measures can be taken into consideration in
with each other. Each top 20 comorbidity was directly
order to prevent the deterioration of health of hyper-
connected to an average of 3.3 other comorbidities tension patients.
and the number of comorbidities between any two
The sex-specific and age-specific detection rates
comorbidities was only approximately one.
of comorbidities described the different risks of
comorbidities in hypertension patients with a differ-
Discussion
ent sex and age range. We found that female hyper-
In our study, we obtained a large collection of tension patients were more likely suffer from osteo-
electronic medical records from 106 prestigious hos-
porosis, while male hypertension patients were more
pitals located in 72 cities in China. The data collection
likely to develop renal insufficiency. Nephropathy,
process was mostly automatic and involved little uremia, and anemia were important risk factors in
human intervention. The automation of data collec-
hypertension patients younger than 39 years, while
http://www.medsci.org
Int. J. Med. Sci. 2016, Vol. 13
CHD, diabetes, hyperlipemia, arteriosclerosis, and medical data collection process need to be executed to
cerebral infarction were high risk factors in older hy-
ensure a more comprehensive data collection.
pertension patients. Those findings can provide
guidelines for the prevention of comorbidities of hy-
Conclusions
pertension. An example of a preventative measure is
In summary, our analysis of comorbidities of
with diabetes, one of the most frequently observed hypertension in China between 2011 and 2013 pro-
comorbidities of hypertension, where reducing sugar
vided an overview of the detection rate of comorbidi-
intake is a common proposal for hypertension pa-
ties among hypertension patients. Variate detection
tients. In addition, kidney disease is the most im-
rates of comorbidities regarding age and sex were
portant risk factor in young hypertension patients. presented, and the co-occurrence relationships among
Therefore, patient's sex and age should be accounted
comorbidities were analyzed. Our findings can sup-
in when proposing prevention measures for hyper-
port doctors and patients to make more specific di-
tension patients.
agnoses and treatment plans by considering patient's
Currently, medically-aided diagnostic technolo-
age, sex and comorbidity conditions. Our results can
gies primarily focus on preliminary statistics and a also increase people's awareness of the comorbidities
probabilistic computing system. In the era of large of hypertension. Further study on hypertension and
amounts of data, network-based recommendation its comorbidities will likely improve the life quality of
technology continues to be developed. The character-
hypertension patients, and be helpful for the preven-
istics of comorbidities that are calculated from a tion of hypertension.
comorbidity network could provide valuable infor-
mation about the relationships between each comor-
bidity pair. Our findings could rapidly promote the
CHD: Coronary Heart Disease; CI: Confidence
development of new diagnostic technologies, not only
for hypertension, but also for other diseases.
In the current study, the high-frequency
Supplementary Material
co-occurrence relationships among comorbidities of Figures S1-S2, Table S1.
hypertension were analyzed and presented by the http://www.medsci.org/v13p0099s1.pdf
comorbidity network. Relationships with a high edge
weight indicated those two comorbidities had a high
co-existing correlation. The core network comprising
This study was funded by National Natural
the top four comorbidities verified a strong
Science Foundation of China (Nos. 91024030,
co-occurring relation and high risk of those four 71025001, 91224008, 91324007) and Important Na-
comorbidities to hypertension patients. Anemia and tional Science & Technology Specific Projects (Nos.
uremia had a relatively lower relevance with comor-
2012ZX10004801, 2013ZX10004218).
bidities in the core network than nephropathy did.
Moreover, arthritis, osteoarthropathy, and gastritis
Competing interests
had a relatively independent morbidity risk with the
core network. In overall, the high-frequency
The authors declared that they had no compet-
co-occurrence relationships among comorbidities ing interests.
could be important for prevention and treatment of References
hypertension and its comorbidities.
1. Gu DF, Reynolds K, Wu XG, Chen J, Duan XF, Muntner P, et al. Prevalence,
There are limitations of this study. First, the de-
awareness, treatment, and control of hypertension in China. Hypertension.
tection rates achieved from different cities had shown
2002; 40: 920-7.
2. Wolf-Maier K, Cooper RS, Banegas JR, Giampaoli S, Hense HW, Joffres M, et
different patterns. Further study, such as spatial
al. Hypertension, prevalence and blood pressure levels in 6 European
analysis, could reveal the reasons for those differ-
countries, Canada, and the United States. JAMA. 2003; 289: 2363-9.
3. He J, Gu DF, Wu XG, Reynolds K, Duan XF, Yao CH, et al. Major causes of
ences. Second, some ambiguous or casually typed
death among men and women in China. N Engl J Med. 2005; 353: 1124-34.
records were ignored due to the insufficiency of the 4. Sheng CS, Liu M, Kang YY, Wei FF, Zhang L, Li GL, et al. Prevalence,
awareness, treatment and control of hypertension in elderly Chinese.
natural language processing techniques that we had
Hypertens Res. 2013; 36: 824-8.
utilized. Adopting more effective text mining tools 5. Al-Tuwijri AA, Al-Rukban MO. Hypertension control and co-morbidities in
primary health care centers in Riyadh. Ann Saudi Med. 2006; 26: 266-71.
might increase the validity of rules that we used and
6. Hirani V, Zaninotto P, Primatesta P. Generalised and abdominal obesity and
the likelihood of finding new rules. Third, some
risk of diabetes, hypertension and hypertension-diabetes co-morbidity in
England. Public Health Nutr. 2008; 11: 521-7.
seemingly unrelated or undetected patient symptoms
7. Wang R, Zhao Y, He X, Ma X, Yan X, Sun Y, et al. Impact of hypertension on
health-related quality of life in a population-based study in Shanghai, China.
might not have been completely and thoroughly rec-
Public Health. 2009; 123: 534-9.
orded in the system. More detailed inspections on the
8. in't Veld AJM. Symptomatic BPH and hypertension: Does comorbidity affect
quality of life? Eur Urol. 1998; 34: 29-36.
http://www.medsci.org
Int. J. Med. Sci. 2016, Vol. 13
9. Aung T, Bisognano JD, Morgan MA. Allergic respiratory disease as a potential
38. Opsahl T, Agneessens F, Skvoretz J. Node centrality in weighted networks:
co-morbidity for hypertension. Cardiol J. 2010; 17: 443-7.
Generalizing degree and shortest paths. Social Networks. 2010; 32: 245-51.
10. Prudenzano MP, Monetti C, Merico L, Cardinali V, Genco S, Lamberti P, et al.
39. Tang CL, Wang WX, Wu X, Wang BH. Effects of average degree on
The comorbidity of migraine and hypertension. A study in a tertiary care
cooperation in networked evolutionary game. EPJB. 2006; 53: 411-5.
headache centre. J Headache Pain. 2005; 6.
40. Fronczak A, Fronczak P, Holyst JA. Average path length in random networks.
11. Dzudie A, Kengne AP, Mbahe S, Menanga A, Kenfack M, Kingue S. Chronic
PhRvE. 2004; 70.
heart failure, selected risk factors and co-morbidities among adults treated for
hypertension in a cardiac referral hospital in Cameroon. Eur J Heart Fail. 2008;
12. Channanath AM, Farran B, Behbehani K, Thanaraj TA. State of Diabetes,
Hypertension, and Comorbidity in Kuwait: Showcasing the Trends as Seen in
Native Versus Expatriate Populations. Diabetes Care. 2013; 36: E75-E.
13. Weiderpass E, Persson I, Adami HO, Magnusson C, Lindgren A, Baron JA.
Body size in different periods of life, diabetes mellitus, hypertension, and risk
of postmenopausal endometrial cancer (Sweden). Cancer Causes Control.
2000; 11: 185-92.
14. Lukas A, Kumbein F, Temml C, Mayer B, Oberbauer R. Body mass index is the
main risk factor for arterial hypertension in young subjects without major
comorbidity. Eur J Clin Invest. 2003; 33: 223-30.
15. Uretsky S, Messerli FH, Bangalore S, Champion A, Cooper-DeHoff RM, Zhou
Q, et al. Obesity paradox in patients with hypertension and coronary artery
disease. Am J Med. 2007; 120: 863-70.
16. Bixler EO, Vgontzas AN, Lin HM, Ten Have T, Leiby BE, Vela-Bueno A, et al.
Association of hypertension and sleep-disordered breathing. Arch Intern Med.
2000; 160: 2289-95.
17. Sarafidis PA, Li S, Chen SC, Collins AJ, Brown WW, Klag MJ, et al.
Hypertension awareness, treatment, and control in chronic kidney disease.
Am J Med. 2008; 121: 332-40.
18. Abougalambou SSI, Abougalambou AS. A study evaluating prevalence of
hypertension and risk factors affecting on blood pressure control among type
2 diabetes patients attending teaching hospital in Malaysia. Diabetes Metab
19. Wu YF, Huxley R, Li LM, Anna V, Xie GQ, Yao CH, et al. Prevalence,
Awareness, Treatment, and Control of Hypertension in China Data from the
China National Nutrition and Health Survey 2002. Circulation. 2008; 118:
20. Wang J, Ning X, Yang L, Lu H, Tu J, Jin W, et al. Trends of hypertension
prevalence, awareness, treatment and control in rural areas of northern China
during 1991-2011. J Hum Hypertens. 2014; 28: 25-31.
21. Schillaci G, Pirro M, Vaudo G, Gemelli F, Marchesi S, Porcellati C, et al.
Prognostic value of the metabolic syndrome in essential hypertension. J Am
Coll Cardiol. 2004; 43: 1817-22.
22. Wong ND, Lopez V, Tang S, Williams GR. Prevalence, treatment, and control
of combined hypertension and hypercholesterolemia in the United States. Am
J Cardiol. 2006; 98: 204-8.
23. Beckett NS, Peters R, Fletcher AE, Staessen JA, Liu LS, Dumitrascu D, et al.
Treatment of hypertension in patients 80 years of age or older. N Engl J Med.
2008; 358: 1887-98.
24. Tanushi H, Dalianis H, Nilsson GH. Calculating prevalence of comorbidity
and comorbidity combinations with diabetes in hospital care in sweden using
a health care record database. 3rd International Workshop on Health
Document Text Mining and Information Analysis 2011, LOUHI 2011, July 6,
2011 - July 6, 2011. Bled, Slovenia: Sun SITE Central Europe CEUR-WS; 2010:
25. Kilander L, Nyman H, Boberg M, Hansson L, Lithell H. Hypertension is
related to cognitive impairment - A 20-year follow-up of 999 men.
Hypertension. 1998; 31: 780-6.
26. Barabasi AL. Network medicine - From obesity to the "Diseasome' . N Engl J
Med. 2007; 357: 404-7.
27. Goh KI, Cusick ME, Valle D, Childs B, Vidal M, Barabasi AL. The human
disease network. Proc Natl Acad Sci U S A. 2007; 104: 8685-90.
28. Hidalgo CA, Blumm N, Barabási A-L, Christakis NA. A Dynamic Network
Approach for the Study of Human Phenotypes. PLoS Comput Biol. 2009; 5:
29. Shi J, Hu M, Shi X, Dai G-Z. Text segmentation based on model LDA. Chin J
Comp. 2008; 31: 1865-73.
30. Fu GH, Kit C, Webster JJ. Chinese word segmentation as morpheme-based
lexical chunking. Inf Sci. 2008; 178: 2282-96.
31. Sanner MF. Python: A programming language for software integration and
development. J Mol Graph Model. 1999; 17: 57-61.
32. Hall M, Frank E, Holmes G, Pfahringer B, Reutemann P, Witten IH. The
WEKA data mining software: an update. ACM SIGKDD explorations
newsletter. 2009; 11: 10-8.
33. Moon TK. The expectation-maximization algorithm. ISPM. 1996; 13: 47-60.
34. Roque FS, Jensen PB, Schmock H, Dalgaard M, Andreatta M, Hansen T, et al.
Using Electronic Patient Records to Discover Disease Correlations and Stratify
Patient Cohorts. PLoS Comput Biol. 2011; 7.
35. Jensen AB, Moseley PL, Oprea TI, Ellesoe SG, Eriksson R, Schmock H, et al.
Temporal disease trajectories condensed from population-wide registry data
covering 6.2 million patients. Nat Commun. 2014; 5: 4022.
36. Bavelas A. Communication patterens in task-oriented groups. J Acoust Soc
Am. 1950; 22: 723-30.
37. Newman MEJ. The structure of scientific collaboration networks. Proc Natl
Acad Sci U S A. 2001; 98: 404-9.
http://www.medsci.org
Source: http://ir.ia.ac.cn/bitstream/173211/11433/1/2016IJMS_Comorbidity%20analysis%20according%20to%20sex%20and%20age%20in%20hypertension%20patients%20in%20China.pdf
Clinical Case Series Adipose Derived Stem and Regenerative Cells for the Treatment of Equine Joint Injuries Vet-Stem, Inc., 12860 Danielson Court, Suite B, Poway, CA 92064 Developmental bone disease, osteochondritis dissecans (OCD), and subchondral bone cysts compromise surface cartilage and the underlying supporting bone. Traumatic injuries to joints and their supporting structures happen frequently to high performance athletes as these are the regions under the greatest stress during intense activity. Whether a horse inherits a tendency towards musculoskeletal disease or acquires an injury, if left unchecked, this may lead to degenerative joint disease, osteoarthritis, eventual joint breakdown, and a reduction in functional career longevity.
Treatment Options for Glioblastoma and other Prepared by Ben A. Williams Glioblastoma Diagnosis, March 30, 1995 Last Updated: March 10, 2014 Copyright 2014 Ben Williams Disclaimer: the information presented here is the opinion of Ben Williams. It is for informational purposes only, do not consider it medical advice. Discuss the