Kmel-journal.org
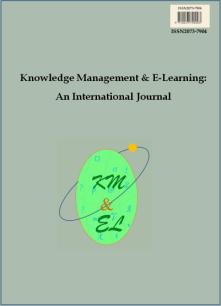
Knowledge Management & E-Learning, Vol.6, No.4. Dec 2014
Knowledge Management & E-Learning
Informing physicians using a situated decision support
system: Disease management for the smart city
Raafat George Saade
Rustam Vahidov
Concordia University, Montreal, Canada
George M. Tsoukas
Alexander Tsoukas
McGill University, Montreal, Canada
Recommended citation:
Saade, R. G., Vahidov, R., Tsoukas, G. M., & Tsoukas, A. (2014).
Informing physicians using a situated decision support system: Disease
management for the smart city. Knowledge Management & E-Learning,
6(4), 472–492.
Knowledge Management & E-Learning, 6(4), 472–492
Informing physicians using a situated decision support
system: Disease management for the smart city
Raafat George Saade*
John Molson School of Business Concordia University, Montreal, Canada E-mail: [email protected]
John Molson School of Business Concordia University, Montreal, Canada E-mail: [email protected]
George M. Tsoukas
Faculty of Medicine McGill University, Montreal, Canada E-mail: [email protected]
Alexander Tsoukas
Faculty of Medicine McGill University, Montreal, Canada E-mail: [email protected]
*Corresponding author
Abstract: We are in the midst of a healthcare paradigm shift driven by the
wide adoption of ubiquitous computing and various modes of information
communications technologies. As a result, cities worldwide are undergoing a
major process of urbanization with ever increasing wealth of sensing
capabilities – hence the Internet of Things (IoT). These trends impose great
pressure on how healthcare is done. This paper describes the design and
implementation of a situated clinical decision support (SCDSS) system, most
appropriate for smart cities. The SCDSS was prototyped and enhanced in a
clinic. The SCDSS was then used in a clinic as well as in a university hospital
centre. In this article, the system's architecture, subcomponents and integrated
workflow are described. The systems' design was the result of a knowledge acquisition process involving interviews with five specialists and testing with 50 patients. The reports (specialist consultation report) generated by the SCDSS were shown to general practitioners who were not able to distinguish them from human specialist reports. We propose a context-aware CDSS and assess its effectiveness in managing a wide medical range of patients. Five different patient cases were identified for analysis. The SCDSS was used to produce draft electronic specialist consultations, which were then compared to
the original specialists' consultations. It was found that the SCDSS-generated consults were of better quality for a number of reasons discussed herein.
Knowledge Management & E-Learning, 6(4), 472–492
SCDSSs have great promise for their use in the clinical environment of smart cities. Valuable insights into the integration and use of situated clinical decision support systems are highlighted and suggestions for future research are given.
Keywords: Clinical workflow; Disease management; Smart cities; Decision
support
Biographical notes: Dr. Raafat George Saade has been teaching in the faculty
since 1998. He obtained his PhD in 1995 (Concordia University) after which he
received the Canadian National Research Council postdoctoral fellowship,
which he completed at McGill University in Montreal. Dr. Saade has published
in journals such as Information & Management, Journal of Information
Technology and Education, Decision Sciences, Decision Support Systems, and
Expert Systems with Applications. His research interests include the
implementation of information systems, the supply chain of digital information
products, and change management.
Dr. Rustam Vahidov is a Professor at the John Molson School of Business at Concordia University. He received his PhD in Decision Sciences from Georgia State University, Atlanta in 2000, his MBA in Decision Sciences from Georgia State University in 1997, and his BSc in Management Information Systems from Azerbaijan State Oil Academy in 1991. His research focuses on Decision Support Systems, Multi-Agent Systems, Fuzzy Logic, Genetic Algorithms, and Neural Networks.
Dr. George M. Tsoukas is a senior endocrinologist at the McGill University Health Centre (MUHC). He received his medical degree from McGill University. Dr. Tsoukas' medical and research discipline include the molecular biology and the endocrinology of bone and diabetes. He has authored numerous papers, particularly in the treatment of Paget's disease of bone. He is a fellow of the Royal Canadian College of Physicians and also a member of a number of professional societies including the American Society for Bone and Mineral Research and the American Heart Association.
Dr. Alexander Tsoukas, MD is a rheumatologist at Division of Rheumatology, Faculty of Medicine, McGill University.
1. Introduction
Functionality of clinical information systems have grown from rudimentary data entry and retrieval on an intra-hospital basis, to real-time data retrieval, multi-user data entry, multi-access data retrieval, knowledge sharing, sophisticated consultation, patient and inter-practice management, competition support, and enhanced decision support. With the advent of the physician workstation, hand-held data entry systems, voice recognition systems, and real-time clinical data retrieval and electronic medical record update, clinical information systems are developing into comprehensive solutions integrating many aspects of the care delivery process. Innovative point-of-care support, such as vital sign monitoring, medication administration monitoring, basic chart maintenance, lab and drug orders administration, and alerting, are reducing labour needs while increasing accuracy and quality through the continuous update of the electronic medical records
(Sittig & Singh, 2010). The development of technology that has led to greater "alerting and protocol support, utilization control, case management, outcome management, and
R. G. Saade et al. (2014)
executive decision support" (Vahidov, Kersten, & Saade, 2014), have enhanced the care delivery process, particularly the decision support aspect of the clinical information system.
Functionality of clinical information systems have grown from rudimentary data
entry and retrieval on an intra-hospital basis, to real-time data retrieval, multi-user data entry, multi-access data retrieval, knowledge sharing, sophisticated consultation, patient and inter-practice management, competition support, and enhanced decision support. With the advent of the physician workstation, hand-held data entry systems, voice recognition systems, and real-time clinical data retrieval and electronic medical record update, clinical information systems are developing into comprehensive solutions integrating many aspects of the care delivery process. Innovative point-of-care support, such as vital sign monitoring, medication administration monitoring, basic chart maintenance, lab and drug orders administration, and alerting, are reducing labour needs while increasing accuracy and quality through the continuous update of the electronic medical records (Sittig & Singh, 2010). The development of technology that has led to
greater "alerting and protocol support, utilization control, case management, outcome management, and executive decision support" (Vahidov, Kersten, & Saade, 2014), have enhanced the care delivery process, particularly the decision support aspect of the clinical information system.
One important type of medical information systems (an advanced application of
electronic health record information systems) includes those targeting decision support for conducting medical diagnosis and disease management (Kastner et al., 2010). ICT
decision support in smart cities is unavoidable. The notion of ‘smart cities' envisions cities with technological infrastructures able to support ambient intelligence. In that sense, the acquisition and use of large data for the development of application to support decision-making capabilities are boundless. A number of major initiatives have taken root
in establishing frameworks for ‘smart cities': MIT (http://cities.media.mit.edu/); European; and IBM. The MIT smart cities framework is part of their media lab and they
have named it ‘City Science'. They categorize the initiative into urban analytics and modeling, incentives and governance, mobility networks, places of living and work, electronic and social networks, and energy networks. The MIT smart cities initiative is environmental-centric, with a small provision of medicine that may fit into their electronic and social networks initiative. The European smart cities initiative includes a smart cities model, ranking, benchmarking and city profiles. Companies such as IBM are taking action to establish themselves as leaders in the smart cities initiatives They have identified a framework mapping important areas in which smart cities can play a key role as follows (Boulos & Al-Shorbaji, 2014; Boulos et al., 2011).
1. Planning and management
a. Public safety b. Government and agency administration c. City planning and operations d. Buildings
2. Infrastructure
a. Energy b. Water c. Transportation
3. People
a. Education
b. Smarter Care
Knowledge Management & E-Learning, 6(4), 472–492
i. Solution for care management
ii. Asset management
iii. Fraud and abuse management for payers
iv. Healthcare asset management
v. Member 360 for healthcare
vi. Solution for healthcare reform
vii. Business analytics for healthcare
viii. Advanced care insights
c. Social programs
In all these ‘smart cities' frameworks, smart medicine lacks representation.
Healthcare in ‘smart cities' should address intelligent ways in doing medicine and not simply the digitizing of patient file. It is this gap that our article attempts to fill. We therefore propose (and demonstrate the benefits) herein, a situated clinical decision support system (SCDSS) as a solution to fill this gap. In the next two sections we elaborate on decision support systems followed by the implementation of a SCDSS.
2. Decision support systems
Decision support systems (DSS) have been traditionally categorized as data-, model-, and knowledge-based. Model-based DSS rely on computational models and algorithms used to calculate optimal solutions to the problems at hand or assess the impact of various candidate decisions on problem criteria. However, medical diagnosis is a complex human process that is difficult to represent in an algorithmic model. Not only does medical diagnosing require the understanding of symptoms, drug-drug interactions, and patient history, the diagnosing process requires knowledge of the fundamental principles of a
diseases' onset and evolution in general and especially as it differs within the general population. Furthermore, the system would have to be (1) updateable to constant changes that accompany the scientific development - a result of the extensive research within the medical field (Ahmadian, 2011), and (2) able to utilize different types of data and medical information (such as signs and symptoms) in order to diagnose an individual. While one patient may have data showing high cholesterol, chest pain, higher blood pressure within an arterial section, and previous heart attack history within the family, another patient may only show high cholesterol and chest pain. While both patients may require a catheterization, the limited data of the second patient may hinder the validity of the diagnosis, and therefore, could lead to the misdiagnosis of the patient (Sintchenko, Iredell, Gilbert, & Colera, 2005).
Furthermore, it is imperative that the diagnosing systems provide explanation for
the generated medical diagnosis. Such capability would make system's decision-making process transparent to the physician. In light of the above requirements we agree that effective decision support in the medical field should primarily rely on knowledge-based systems (Write et al., 2009) incorporating relevant models, tools and techniques.
There is a large body of literature available on decision support system
applications in many fields. However, relatively few of them are in the field of healthcare and even less in the clinical practice of medicine. We group decision support systems (DSS) used in healthcare into six types: (1) Acute care, (2) Disease management, (3) Educational, (4) Laboratory systems, (5) Medical imaging and (6) Quality assurance and administration. "Intelligent Decision Support System" as a generic term has been used to cover numerous types of intelligent systems that can be applied in the medical field. Clinicians see those systems as black boxes and the security of the medical data used
R. G. Saade et al. (2014)
requires that they be thoroughly evaluated, before they are acceptable (Smith, Nugent, & McClean, 2003). Intelligent medical decision support systems can support diagnostic and disease management processes. Examples of medical decision support systems in use today can be found in This table classifies the DSSs into those that have been used in the clinic, web-based, knowledge-based and used for information management.
Use of computer assisted decision support systems in the clinical practice has
been reported to facilitate better patient care (Kastner et al., 2010). A survey of medical DSS applications has revealed that clinical DSS have improved practitioner performance in 64% of studies, including diagnosis support, reminder systems, disease management support, and drug dosing and prescription support (Garg et al., 2005). As far back as two decades ago, (Berner et al., 1994) published the results of a study in which four commercially available medical diagnostic systems were challenged to diagnose a series of 105 patients each of whom had been referred to a consultant and in which of whom a diagnosis had been established. The programs studied included Dxplain, Iliad, Meditel and QMR. At that time, the proportion of correct diagnosis ranged from 52% to 71% and the relevant diagnoses ranged from 19% to 37%. Looking back, these results can be considered good. Since that time information technology has improved exponentially and therefore it is expected that these numbers would be much higher today.
Table 1
Different categories of decision support systems
Description
Clinical Feedback
Category
Drug Alerts
Objectives: decrease rate of medication
Reported to be effective.
Aid in clinical diagnosis
Possible benefits on relatively easier clinical cases. The role of computer-aided diagnostics remains open to debate.
Electronic assistance for practitioner and
Can lead to a favorable change in
patient decision-making.
clinical behavior.
Patient data is stored in electronic format.
Computerization in practice.
Patient Records Lab Alerts
Ordering and interpretation of lab tests.
Reported to be effective.
Helps speed up work flow in clinics
Has been used to measure user
perception/attitudes to new technology or system.
Reminders used to reduce errors.
Reported to be effective.
Information provided after a given
May potentially modify physicians
test is ordered.
practice profiles.
Effective disease management solutions should ideally be organically integrated
into the workflow of healthcare providers, and should follow clinical practice guidelines. Such guidelines that define what steps are necessary in order to ensure quality care provision can be separated into decisions, actions, and processes (Fossu, Alexander, Ehnfor, & Ehrenberg, 2011). The decision model would include selection of relevant variables with differing weights of importance, of diagnosis, and consideration of alternative diagnoses. By utilizing such a system, the patient and the physician would
become "collaborators" in managing what information is relevant and which result to act upon. Furthermore, the action model would specify the actions that need to be performed.
Knowledge Management & E-Learning, 6(4), 472–492
These actions would include the specification of type of action and temporal limitation (i.e. take dose for three months) through the standardized medical terminology available. Finally, the process model would organize actions sequentially and hierarchically in order to determine which actions are crucial to the care process and in what order the care should be delivered (Fossu, Alexander, Ehnfor, & Ehrenberg, 2011). The creation of clinical practice guidelines is necessary in order to have a template with which the system may prescribe diagnoses, actions, and processes. From our literature review, we can identify (see Table 1) eight major categories by which decision support systems were used in a clinical environment.
In this paper, we present a clinical decision support system for the assessment of
patients with osteoporosis based on the situated decision support (Vahidov & Kersten, 2004, Vahidov, Kersten, & Saade, 2014) approach (SDSS). Situated DSS model is based on the principle of combining decision support with maintaining intimate links with the problem/knowledge domain, as opposed to a classical stand-alone DSS approach. The purpose of this work is to present the design and test a decision support system situated in a clinical environment. This in effect has two dimensions: (1) the ability of the SCDSS to accurately assess a patient and (2) the effectiveness of the SCDSS in differentiating the
patients' assessment due to different medical conditions.
3. The situated clinical DSS
The SCDSS in this study was developed to investigate the applicability of context-aware (hence situated) DSSs in the management of diseases, medical conditions and disorders. Within the context of medical care, the situated decision support system framework developed in response to the need for integration of the traditional DSS into the organizational workflow (Vahidov, Kersten, & Saade, 2014) can be used to account for the context in managing patient in the clinical workflow. Initially the focus of DSS research and development was on generic problem-solving activities. It was primarily
used as a "stand alone" application outside of business work processes. Moreover, the traditional DSS mostly focused on single-shot decisions, without integrating feedback assessment, the context of the environment it is being used for, and corrective actions. Situated DSS model envisages tight integration of active decision support with the problem environment and on-going monitoring of situation with the possibility of intervention. The key operative term is active where the SDSS interacts with all participants: patient, secretary, nurse, and doctor.
The conceptual model of the SDSS can be viewed in Fig. 1. The inner-most layer
is the DSS manager. The middle layer entails the key components for situating the DSS: the sensory system, which includes ‘sensors' that solicit/receive health information from
the patient and ‘effectors' that send information/feedback to the patient. The outer-most layer includes the patient environment, which could be either virtual or physical, or it can span across both.
Generally speaking, situating the DSS necessitates the addition of at least two key
capabilities: (i) the capability to access the health/medical conditions (sensors), and (ii) the capability to change the environment (effectors) surrounding those conditions. Sensors, effectors (together with the manager), and active user interface comprise the generic SDSS. The Manager is composed of the traditional DSS components (i.e.
database, models, and knowledge base) relevant to a problem domain and an "active" component: the DSS inference. The inclusion of the inference allows SDSSs to be active even in the absence of the decision maker and capable of performing certain tasks
R. G. Saade et al. (2014)
autonomously (e.g. contacting the patient, preparing the medical DSS for interaction prior
to the patient's request, and even making decisions within the limits of medical best practices and recommendations). To this end, the manager requires a knowledge base containing business rules.
Fig. 1. The proposed situated decision support system
Sensors and effectors are the tools used by a DSS to interact with the patient
environment and engage in different activities required to implement a decision. Implementation of the medical-related decisions primarily involves carrying out the decisions, but it may also entail planning activities, monitoring of execution, reviewing, and negotiating behavioral changes, if necessary. As such, the effectors may also produce reports, generate alerts, send reminders, and perform other relevant actions.
The situated DSS model has been applied to various domains, including
production load management (Hu & Vahidov, 2011), personal finance management (Vahidov & He, 2009), automated negotiations (Vahidov, 2007), service-level agreement negotiations (Vahidov & Neuman, 2008), and project-driven supply chains (Conte & Vahidov, 2008). The management of diseases with context-aware systems is necessary. Osteoporosis management, an important problem, has recently been addressed via a decision support concept (Kastner et al., 2010). However, this concept was not developed into a full system and was mainly paper-based.
The purpose of the present work was to develop a SDSS for the management of
osteoporosis. This was done by obtaining information from medical specialists on how they manage osteoporosis and elicits information to specify system functionalities and features. We also observed the clinical environment for a week in order to understand the
Knowledge Management & E-Learning, 6(4), 472–492
context of its operation and patient management. Based on the clinical workflows and context-specific operation, we adapted the framework for situated DSS and developed the Situated Clinical DSS - SCDSS proposed in this article. The primary outcome of the SCDSS was to facilitate the capture of high quality and relevant data, and produce a meaningful physician oriented expert consultation.
We further elaborate on the use of the different SCDSS components. A number of
distinct applications that can be viewed as sensors or effectors by which they allow the different users to interact with their patient environment:
Drug intake alert; Lab tests and medical diagnostics engine; Electronic consultations; Referrals; Dietetics management; Exercise management;
Example 1: Drug Intake Alert
A list of prescribed drugs is specified along with the details of administration including quantity, time of day, before or after meals, or even specific times etc. The system can therefore inform the user when the patient needs to administer the drug and when someone else needs to administer the drug. The information can be conveyed to the user via a cell phone and be immediately informed to take proper action. The system can also request from the patient to respond with specific data once the drug has been administered. The system can therefore record when the drugs are actually taken and build a database of drug usage that could be utilized later for statistical purposes. The system therefore monitors and evaluates whether the patient is following the doctors advice and the suggested regimen for most effectiveness of the drug.
Example 2: Test-Engine Configurator
In general, very few people are aware of the tests they need to undergo for proper management of their health, the time at which they need to take it and the frequency. To that effect, the test engine configurator identifies for the patient the tests he/she needs to
do at a specific point in time and based on their historical profile in the system's database. Such tests may include prostate exams, blood workout, breast exam, and more specific to osteoporosis, physical exam, bone mineral density exam and home safety evaluation.
Data acquisition and interpretation; Family medical; Signs engine; Symptoms engine; Fracture event management; Vital signs monitoring system;
Example 1: Tests Results Data Acquisition System
This function, which complements its web equivalent, serves as a regular point of health-related data entry. It consists in a simple entry form that lets users enter specific day-to-day information. Initially, this module might only accept numeric information that can be immediately interpreted by the system: Glucose level, Blood pressure, Cholesterol levels LDL, HDLTemperature, etc.
Example 2: Symtoms/Signs Query Engine
This engine offers a quick reference card of symptoms of various conditions and diseases. This engine communicates with the inference-engine, the knowledge base and the
patient's file to make decisions on feedback to patient and notification to the patient's doctor. This real-time patient management maximizes the value of medical information and time for both patients and physicians. In the osteoporosis context, if a female patient
R. G. Saade et al. (2014)
enters in the system that she is feeling pain in her bones, then the system will respond with information indicating that pain in bones are not an indication of osteoporosis with links to resources that actually can provide information about it. On the other hand, if a bone densitometry is done and the results were entered; the g/cm2 will be interpreted in the system and in the case that this shows low bone mass, the system will automatically notify the physician and schedule an appointment for the patient for treatment.
4. Implementation of SCDSS
The SCDSS was developed over a period of two years. It was developed using the following knowledge engineering steps: (1) Interview specialists using a cognitive simulation approach where the specialists were asked to walk us through a patient encounter. The first account of the encounter was logged and documented. (2) A second interview session was held at a later time where specialists were asked to recount the same encounter he/she did in the first interview, however this time the knowledge
engineer would interrupt the specialist with ‘what-if' cases. (3) All results were then integrated and reconciled. Some of the primary challenges of applying this approach to the medical field are: Disagreement between specialists on medical details; difficulty of specialists to reflect and recount their cognition (tacit knowledge) on how they manage
their medical practice and specialists' lack of understanding of data, information and information processing.
Once everything was reconciled and the knowledge base frozen, the SCDSS
subsystems were developed. The report generator was designed and developed based on 50 cases from one specialist reports. These reports were analyzed for structure, content and style and were then aligned with our knowledge base. The report generator parses the entire report sentences to the word level, compares data entered by the user to the knowledge base and then captures knowledge fragments from the knowledge base and reconstructs the report.
The inference engine of the SCDSS is composed of screening, assessing and
reporting components. En example of the logic embedded in the system is shown in Fig. 2. The screening component identifies whether the patient is new or is already in the system. If the patient is new, then the system opens a basic medical file while if the patient already exists then the system prompts for follow-up questions. The assessment component uses the Subjective, Objective, Assessment, and Plan (SOAP) approach to medical management: The subjective description of the patient's reasons for the visit; the objective findings including physical examination and laboratory; the assessment by the knowledge base of the system; and the plan of action proposed by the system. To integrate the SCDSS into the clinical environment the specialist uses the problem-oriented mode, which enables him or her to further elaborate on the patient and then to assign additional clinical information to the final assessment and plan.
The medical-record module of the SCDSS provides the specialist with functions
that use or augment the data analysis capabilities in the computer-based record to, for example, monitor drug interaction and contraindications, access practice guidelines, summarize patient histories, monitor risk profiles such as fracture risks, screen patients such as women eligible for different treatments, or conduct follow-up.
Knowledge Management & E-Learning, 6(4), 472–492
Fig. 2. SCDSS embedded logic
A patient enters the system by logging in using the assigned username and
password. If the patient is a new patient then he/she is prompted with question to complete a basic patient file. If the patient already exists in the system, then the SCDSS will recognize him/her and prompt him/her with a follow-up set of questions based on their last visit. In either case, the SCDSS will generate an ‘eSession' identifying the set of important questions that need to be prompted to the patient and that are pertinent to the reason of the visit.
Following this initial session screening, the ‘eSoap' approach is executed by the
system. The patient, nurse, and doctor interact with the SCDSS at the appropriate times
and a draft ‘econsult' report is generated. At the end of the day, the specialist enters the SCDSS via a secure connection and views a list of all the patients that have been assessed for the day. The specialists can then verify, edit and approve the reports and approve the system to release two ‘econsult' reports: one for the referring physician and one for the patient, with customized information appropriate to the physician and to the patient.
The knowledge base includes close to 250 inter-related questions, over 4000
words of medical terminology, and over 200 rules.
5. Discussion and analysis of results
As previously mentioned, the system was developed based on the reports that a specialist produced for 50 patients. As part of the pilot, the SCDSS was utilized in a clinic for 45 patients. In this paper we present the econsult generated by the SCDSS for five patients with the different levels of osteoporosis conditions (given in Table 2, a to e (see Appendix)). The goal is to assess the ability of the SCDSS to provide acceptable consultations and compare them to actual (manual-based) ones. Table 2 also provides the original physician consultations side-by-side with the SCDSS ones for comparisons. Analysis on the text was also performed and reported.
For the purpose of this study, five significantly different osteoporosis patients
(which we refer to them as Patient X1 to X5) are selected to test the SCDSS for its capability of assessing the patient and producing different expert consultation reports, which are at least equivalent to that generated by the specialist. Considering the original
specialist's reports, the five patients presented in Table 2 have osteoporosis – X1 has
R. G. Saade et al. (2014)
severe osteoporosis. All patients are older than 65 years where X1's age is not reported and X5 is 85 years old. The lengths of the reports vary along with the categories of
medical information reported. We noticed that some ‘casual' language had been used such as in patient X5 – ‘sitting duck'.
It is evident from Table 2, a to e, that the SCDSS consults have a number of
significant advantages: (1) consistency of information reported; (2) reporting on only relevant information and data; (3) standardization of the categories to report on; (4) integration of medical associations and findings as interpreted by the knowledge base and inference; (5) completeness of the report; and (6) integration of impressions by the specialist. It is important to note at this point that the consult generated by the SCDSS is labeled as draft whereby editing is made possible for the specialist followed by final approval before the report is released.
There are two measures, as mentioned earlier, that we are seeking to evaluate
from these results produced by the SCDSS: (1) its ability to produce an accurate consultation and (2) its effectiveness in differentiating between different medical cases.
Table 2 which present the original specialist's consultation and the SCDSS-generated econsult side-by-side (for five patients), shows that the SCDSS econsult passes both evaluations and may be considered even superior from the original manual-based specialist report – as we demonstrate further the analyzed reports (Tables 3 to 7).
Tables 3 and 4 present the content analysis of specialist's consultations in terms
of what information and how much of it (sentences) is included (for ten patients), respectively. We notice that in Table 3, calcium total, creatinine, alkaline phosphatase and TSH are included in at least eight of the ten patients, indicating that the specialist considered in this study is focused on the markers for his/her decision making process. Table 4 on the other hand, shows the number of sentences written on a specific medical subject such as signs and symptoms with the total number of words given in the last
column. ‘Signs' was the most consistently reported medical category while ‘gynecology'
was the least. Overall the variation between the consultations' amount of content is large varying from 198 to 523 words.
We continued our text analysis further by evaluating the quality of the phrasing in
terms of errors, and awkward. This was done by counting the number of sentences that had errors and that were awkward. Table 5 shows that only one of the consultations had zero errors and the rest had either one or two. In terms of awkward phrasing, six consultations had only one while the rest had two or three. What is interesting to note and as indicated in Table 5, that none of the consultations included any graphs or images.
Tables 6 and 7 present some comparative data showing the differences between
the specialist's consultation and the SCDSS-generated econsult for the five patients presented in Table 2, in terms of word count and basic descriptive statistics, respectively. A negative difference in the tables imply the SCDSS less that the specialist consultation, and vise versa. In most cases, the word count, minimum, maximum and average are greater in the SCDSS case with an average difference of 26%. What this tells us is that, considering that the medical knowledge and logic in the SCDSS is obtained from the specialists, the original specialist consults in this study are not adequate in terms of reporting and analyzing patient information. Table 6 also confirms our previous text analysis, showing that the variation of word count by is 32% for specialist consultations and 13% for SCDSS-generated econsult. This is a clear and important indication for the SCDSS providing consistent and comparable reports across different patients and with each patient over time.
Knowledge Management & E-Learning, 6(4), 472–492
Table 3
Content analysis of specialist consultations
1: Personal fracture history; 2: Family fracture history; 3: Calcium total; 4: Calcium ionized; 5: Creatinine; 6: Phosphate; 7: Alkaline phosphatase; 8: TSH; 9: PTH; 10: C-telopeptide; 11: Osteocalcin; 12: Medication
Table 4
Qualitative analysis of specialist consultation
1: Signs; 2: Symptoms; 3: Gynecology; 4: Treatment; 5: Follow-up; 6: Interpretation; 7: No. of words
Table 5
Assessment of content quality of specialist consultations
1: Recommendations; 2: Errors; 3: Awkward phrasing; 4: Graphs; 5: No. of paragraphs; 6: No. of pages; Scale used: 1=None; 2=Blurb; 3=One Sentence; 4=Two sentences; and 5=More than two sentences
R. G. Saade et al. (2014)
Table 6
Comparative, with and without SCDSS, word count
Specialist
Difference
254
320
26%
Table 7
Basic statistics, with and without SCDSS
Specialist
Difference
Variation from mean
Fig. 3. Information effectiveness
Fig. 3 depicts the information provided by the specialist as they relate to the
number of words utilized. This relationship provides insight into the quality of the medical information presented. Of course, one would expect that the specialist consultation would include the right amount of medical knowledge, information and data to explain on the rationalization process for the patient health management and support the decisions made. Of course, the quality is questioned when, as shown in Fig. 3, we find two consults with close to 200 words used in each, and such that one provides ten pieces of information while the other only one. We acknowledge that specialists would want to minimize unnecessary information, however, a certain level of rationalization is important and minimal variation would be desirable. The potential of the SCDSS is exactly targeted to address these issues and help specialists in reducing their time to produce a consultation while at the same time maintain an acceptable level of variability and consistency across the consultations.
Knowledge Management & E-Learning, 6(4), 472–492
6. Conclusions
This paper has presented our experience with the integration of a decision support system for the assessment of patients with osteoporosis in a clinical environment. The architecture and working principles of the decision support system as it is situated in the clinical environment were discussed and described. The effectiveness of the SCDSS in generating an electronic consultation is demonstrated and the quality of the consultations were compared to those from a specialist (manually produced).
As mentioned earlier in this article, decision support systems for disease
management is a subset of the ehealth paradigm, and are few and dispersed. The ehealth paradigm in smart cities remains primarily centered around capturing patient data in an electronic record and connecting lab results to physicians, although to a lesser extent. Although the definition of smart cities has not been agreed upon yet, and that its interpretation remains vague, a common and recent definition (Perez-Martinez, Martinez-Balleste, & Solanas, 2013; Solanas et al., 2014) goes as follows:
"Smart cities are cities strongly founded on information and communication technologies that invest in human and social capital to improve the quality of life of their citizens by fostering economic growth, participatory governance, wise management of resources, sustainability, and efficient mobility, whilst they
guarantee the privacy and security of the citizens."
Other definitions still address smart cities in terms of people (citizens) and
technologies (mobile, web, etc.). In the context of ehealth, this definition does not seem adequate. From 1997 to 2006 we engaged in a number of large scale web-based medical projects, interviewed over 60 specialists and a number of general practitioners, and developed a number of intelligent medical systems for disease management. The representation of medical knowledge, which is primarily tacit, was the most complex. Today, with new technologies, this medical knowledge can be represented and encoded into intelligent applications, with great advantages. The application used in this study is the third time it has been programmed from scratch. At the same time, a number of initiatives from government and other institutions were funded and failed.
From our experience, smart cities (at least in healthcare), are not smart because
they capture large amounts of data, nor due to the increase of technologies in the market. The issue of smart cities is more fundamental and entails the integration of the tacit knowledge and common practice into the information technologies. This is only done by collaboration of the IT industry with the people. Let us consider the context in Canada: the needs of the patient, physician, general practitioner, institutions and government are all in conflict. Reminders, journals, scheduling, recommendations, drug administration management are all patient-centric tools but hardly contributes to the smart city.
The future of medicine in smart cities should be driven by knowledge
management systems that integrate tacit knowledge, information technologies, and human stages from birth to death. From the moment a baby is born, it is registered into the SCDSS which already contain all the medical information of parents and
grandparents. The SCDSS follows the baby's medical needs as they arise and provides relevant information to the parents, latest research to specialists, advice to the general practitioner, opens a collaborative forum between the specialist and the baby's doctor, establishes connection with insurance company and manages the general communication between all stakeholders. The data is aggregated, summarized and synthesized hospitals and clinics who can use it for short term resources planning. The paradigm is not as it is today and necessitates an alteration of state-of-mind. The new state-of-mind entails the
R. G. Saade et al. (2014)
proper informing of people and the integration of tacit knowledge in knowledge management systems designed to help people make informed decisions and communicate adequately.
In general, it seems that governments do not have the genuine drive to improve
medicine. Institutions continue to compromise patient health for cost savings, medical specialists have no trust in computerized systems for medicine, general practitioners have not motivation to try new methods, and patients increasingly use Internet sources for medical information and self-diagnoses, thereby creating even more problems for themselves as well as their doctors. All this is not conducive to smart cities. The solution does not lie in the infrastructure nor in the technologies, but rather in the people. As a science, we know what needs to be done and how to do it. Everybody is aware and accepts the advantages. The solution lies in engaging the people in the development of such systems from the start.
The research work demonstrates the feasibility, potential and benefits of
integrating (embedding or situating) a decision support system into the clinical environment. The SCDSS was shown to have potential as an aid to a specialist assessing patients with osteoporosis. The system saves time by streamlining the clinical process and reducing the amount of administrative work. The SCDSS also puts the disease in a focused dynamic setting where the patient learns about the disease and its management
and the patient's primary care physician obtains a comprehensive, consistent and
acceptable quality report. This physician's report is put in the context of academic medicine following most recent scientific publications on this disease.
The difference between the specialist consultation and the SCDSS-generated
econsult, from a medical perspective is significant, with the exception for detailed findings on physical examination, which the specialist may, inserts after the econsult is generated. Thus, the system offers a rapid way of achieving high quality and consistent consultation.
As a follow-up to testing the SCDSS methodology for osteoporosis and as
presented here-in, we joined another team in a hospital running an allergy center. Lessons learned from this experience were applied successfully to the management of allergies – a more complex condition. In this case, the SCDSS was used in a hospital center on an iPAD. With that, we also report that the outcomes in this article and the model proposed and implemented is reproducible.
References
Ahmadian, M. (2011). Factors influencing women's participation in breast cancer
prevention program in Tehran, Iran. Doctoral Research, Universiti Putra Malaysia.
Berner, E. S., Webster, G. D., Shugerman, A. A., Jackson, J. R., Algina, J., Baker, A. L.,
Ball, E. V., Cobbs, C. G., Dennis, V. W., & Frenkel, E. P. (1994). Performance of four computer-based diagnostic systems. The New England Journal of Medicine 330(25), 1792–1796.
Boulos, M. N. K., & Al-Shorbaji, N. M. (2014). On the internet of things, smart cities and
the WHO healthy cities. International Journal of Health Geographics, 13: 10.
Boulos, M. N. K., Resch, B., Crowley, D. N., Breslin, J. G., Sohn, G., Burtner, R., Pike
W. A., Jezirski, E., & Chuang, K. Y. S. (2011). Crowdsourcing, citizen sensing and sensor web technologies for public and environmental health surveillance and crisis management: trends, OGC strandards and application examples. International Journal of Health Geographics, 10: 67.
Knowledge Management & E-Learning, 6(4), 472–492
Conte, G. E., & Vahidov, R. (2008). Architect's decision station and its integration with
project-driven supply chains. In Proceeding of 7th European Conference on Product and Process Modelling. Sophia Antiplois, France.
Fossu, M., Alexander, G. L., Ehnfor, M., & Ehrenberg, A. (2011). Effects of a
computerized decision support system on pressure ulcers and malnutrition in nursing homes for elderly. International Journal of Medical Informatics, 80(9), 607–617.
Garg, A. X., Adhikari, N. K. J., McDonald, H., Rosas-Arellano, M. P., Devereaux, P. J.,
Beyene, J., Sam, J., & Haynes, R. B. (2005). Effects of computerized clinical decision support systems on practitioner performance and patient outcomes. The Journal of the American Medical Association, 293(10), 1223–1238.
Hu, H., & Vahidov, R. (2011). A framework for situated distributed decision support.
Journal of Computer Information Systems, 51(3), 29–37.
Kastner, M., Li, J., Lottridge, D., Marquez, C., Newton, D., & Straus, S. (2010).
Development of a prototype clinical decision support tool for osteoporosis disease management: A qualitative study of focus groups. BMC Medical Informatics and Decision Making, 10: 40.
Perez-Martinez, P. A., Martinez-Balleste, A., & Solanas, A. (2013). Privacy in smart
cities: A case study of smart public parking. In Proceeding of 3rd Intl. Conf. Pervasive Embedded Computing and Communication Systems (pp. 55–59).
Sintchenko, V., Iredell, R. J., Gilbert, L. G., & Colera, E. (2005). Handheld computer-
based decision support reduces patient length of stay and antibiotic prescribing in critical care. Journal of the American Medical Informatics Association, 12(4), 398–402.
Sittig, F. D., & Singh, H. (2010). A new sociotechnical model for studying health
information technology in complex adaptive healthcare systems. BMJ Quality & Safety, 19(3), 68–74.
Smith, A. E., Nugent, C. D., & McClean, S. I. (2003). Evaluation of inherent
performance of intelligent medical decision support systems: utilizing neural networks as an example. Artificial Intelligence in Medicine, 27, 1–27.
Solanas, A., Patsakis, C., Conti, M., Vlachos, S. I., Ramos, V., Falcone, F., Postolache,
O., Perez-Martinez, P., Di Peitro, R., Perrea, D., & Martinez-Balleste, A. (2014). Smart health: A context aware health paradigm within smart cities. IEEE Communications Magazine, 52, 74–81.
Vahidov, R. (2007). Situated decision support approach for managing multiple
negotiations. Lecture Notes in Business Information Processing, 2, 179–189.
Vahidov, R., & He, X. (2009). Situated DSS for personal finance management: Design
and evaluation. Information & Management, 46(8), 453–462.
Vahidov, R., & Kersten, G. E. (2004). Decision station: Situating decision support
systems. Decision Support Systems, 38(2), 283–303.
Vahidov, R., Kersten, G., & Saade, R. (2014). An experimental study of software agent
negotiations with humans. Decision Support Systems, 66, 135–145.
Vahidov, R., & Neumann, D. (2008). Situated decision support for service level
agreement negotiations. Paper presented at the 41st Hawaii International Conference on System Sciences (HICSS), Waikoloa, Big Island, Hawaii.
Write, A., Sitlig, D. F., Ash, J. S., Sharma, S., Pang, J. E., & Middleton, B. (2009).
Clinical decision support capabilities of commercially available clinical information systems. Journal of the American Medical Informatics Association, 16(5), 637–644.
R. G. Saade et al. (2014)
Appendix
Table 2a
Specialist report for patient X1
Date: 6/25/2004
Patient: Patient X1
Date seen: 6/25/2004
Thank you for allowing me to see this diabetic, 65 year old lady. Her diabetes was diagnosed
ten years ago and treated with diet alone. Her origins are from Laconia, 33 years in Canada.
She worked mainly as an operator. Married with five children, her oldest was born in 1951
Thank you for allowing us to assess your patient Ms. X1. Ms. X1, age 76 has a family
and her youngest in 1962.
history of Osteoporosis.
As far as her osteoporosis history is concerned, there is no family history of osteoporosis.
History:
She complains of chronic low back pains and pain in the long bones. Her children we re
Ms. X1 has no T-Score value registered before the last test. Based on the latest DEXA
breast fed 3-6 months each. There is no history of fractures. Mrs. X hates milk and she does
dated 5/14/2004 3:49:17 PM the Tscore of the spine was -2.2 and that of the hip was -
not eat cheese. This was recently reinforced upon her because of the high cholesterol. For the
2.7. Ms. X1 is taking Calcium pills, 500mg, once a day, Fosamax, 70mg once a
past year she has been taking oral calcium about 500mg a day.
week. There is some pain reported in the bones.
Ms. X1 does not suffer from any disease in the past and presently suffers from Arthritis or
A very important event in the history of her illness is that in 1969, she underwent a total
pain in the joints, Rheumatoid arthritis, Diabetes,
abdominal hysterectomy for fibroids and an oophorectomy was also carried out at the same
The patient is post-menopausal. Her menarche was at age 10 and menopause was at 42.
time. This was followed by severe menopausal symptoms. She was given hormones
intermittently but did not actually given proper therapy. The DPX carried out by you is quite
Physical Examination:
shocking and BMD is 0,749. This puts her at a very high risk for fracture and she is almost -
Ms. X1 is 1.52m tall and weighs 66kg. This gives a Body Mass Index (BMI) equal to
4 z-points below the normal. The hip is not as bad, perhaps even normal for her age, at
29. Standing upright with the back against the wall, Ms. X1 was able to have her back
and shoulders firmly on the wall. When asked to bend down and touch her toes, the
patient reached to the ankles (level 2), and reported Strong pain in the upper chest, strong
Physical examination:
pain in the lower chest.
Reveals a pleasant lady in no distress
BP 130/70 Pulse 80
Laboratory Findings:
Calcium Level is Normal; Protein Electrophoresis is Normal; Alkaline Phosphatase is
Examination of her thyroid gland shows it to be mildly enlarged and lumpy bumpy
Normal; and Thyroid Stimulating Hormone is Normal.
There is a moderate dose of kyphosis and painful lateral movements of the cervical spine
Tender over L3-L4 area
Impression:
DTR's are unremarkable
Ms. X1 has Osteoporosis in the spine and Severe Osteopenia in the hip.
She is also tender over the pretibial areas
Impression:
She should have physical exam, dietary history, exercise assessment, fall assessment. She
This lady has severe osteoporosis.
should be very careful not to trip and fall, hence avoid fracture. It is important that Ms. X1
Ideally she should be on Calcimar injections, however, she refuses and for this reason I will
follow a calcium and vitamin D rich diet and exercise regularly. It is recommended that
put her on Premarin 0.625 daily along with Rocaltrol 0.25ug daily. Once we will stabilize
this patient take Calcium pills, 500mg, twice a day, Vitamin D pills, 800 U, once a day,
her a bit we will give her cyclical diphosphonate as well.
and make another blood test, dexa after 1 years.
Secialist, MD, FRCP(C)
Dr. Z, MD, Osteoporosis Specialist
Knowledge Management & E-Learning, 6(4), 472–492
Table 2b
Specialist report for patient X2
Date: 6/25/2004
Patient: Patient X2
As you know, X2 has osteoporosis. This is based on the latest DEXA. The t-score of the
Date seen: 6/25/2004
spine was -3.4 and that of the hip was -2.5. The patient is taking calcium 1g per day,
Fosamax 10mg per day, and vitamin D. There are no specific symptoms except for a dull
ache in the long bones.
In addition to the bone problems, the patient has the following medical conditions: chronic
Thank you for allowing us to assess your patient Ms. X2. Ms. X2, age 71 has a family
anxiety, high blood pressure and cholesterolemia. She is on a diet and is taking the following
history of Osteoporosis(Myself).
medications: Fluvoxamine 50mg qhs, Tiazac 180mg die, Clonazepam 2mg and Losec 10mg
History:
Ms. X2 has no T-Score value registered before the last test. Based on the latest DEXA
Physical Examination: Weight 145 lbs
Height 5'2" BP 170/110
dated 5/17/2004 1:56:56 AM the Tscore of the spine was -2.5 and that of the hip was -
CVS exam – loud S2, no gallops, no evidence of LVH. There are no findings related to the
3.4. Ms. X2 is taking Calcium pills, 500mg, once a day, Vitamin D pills, 400 U, once a
neck. There are no nodes and the thyroid is not felt. The spine is flexible and non tender. No
day, Fosamax, 10mg daily, . There is some pain reported in the bones.
bone tenderness in the extremities. Straight leg raising is normal.
Ms. X2 does not suffer from any disease in the past and presently suffers from Arthritis or
pain in the joints, Rheumatoid arthritis,
Laboratory Findings: PTH, TSH, and calcium are normal. Total cholesterol 6,62.
The patient is post-menopausal. Her menarche was at age 52 and menopause was at 12.
Impression: Osteoporosis, cholesterolemia, anxiety, poorly controlled hypertension
Physical Examination:
Ms. X2 is 1.575m tall and weighs 59kg. This gives a Body Mass Index (BMI) equal to
Recommendation: I do not think that this patient is doing enough to fight her osteoporosis.
24. Standing upright with the back against the wall, Ms. X2 was able to have her back
There should be more exercise, a healthier diet, and strict adherence to taking the
and shoulders firmly on the wall. When asked to bend down and touch her toes, the
medications. I would like to see her again in six months. Please take care of the hypertension
patient reached to the ankles (level 2), and reported.
and dyslipidemia.
Laboratory Findings:
Merry Christmas,
Calcium Level is Normal; Protein Electrophoresis is Normal; Alkaline Phosphatase is
Normal; and Thyroid Stimulating Hormone is Normal.
Impression:
Specialist, MD, FRCP(C)
Ms. X2 has Advanced Osteoporosis in the spine and Severe Osteopenia in the hip.
Recommendation:
She should have physical exam, dietary history, exercise asessment, fall assessment.She
should be very careful not to trip and fall, hence avoid fracture. It is important that Ms. X2
follow a calcium and vitamin D rich diet and exercise regularly. It is recommended that
this patient take Actonel, 35mg daily, and make another blood test, dexa after 0.5 years.
Dr. Z, MD, Osteoporosis Specialist
R. G. Saade et al. (2014)
Table 2c
Specialist report for patient X3
Date: 6/25/2004
Patient: Patient X3
Date seen: 6/25/2004
Thank you for allowing us to assess your patient Ms. X3. Ms. X3, age 77 has a family history of Osteoporosis.
Thank you for sending this lady back again for assessment. As you know she is 71 years old
and has been known to have osteoporosis for a number of years now.
History:
Ms. X3 has no T-Score value registered before the last test. Based on the latest DEXA
dated 4/30/2004 4:01:38 PM the Tscore of the spine was -2.1 and that of the hip was -
The problem with Mrs. X3 is that she is very stubborn and she refuses to take her
5. Ms. X3 is taking Calcium pills, 500mg, once a day, Vitamin D pills, 800 U, once a day, Fosamax, 10mg daily, . There is no pain reported in any of the bones.
medications. Originally started on calcium and vitamin D, her bone density did not increase
Ms. X3 does not suffer from any disease in the past and presently suffers from Arthritis or
and for this reason, last year was given Fosamax. However, because of the cost of the medication, she stopped it. Presently, the patient complains of a constant dull backache. The
pain in the joints, Rheumatoid arthritis, The patient is post-menopausal. Her menarche was at age 13 and menopause was at 50.
patient has not had any recent history of fractures.
Physical Examination:
Ms. X3 is 1.54m tall and weighs 57kg. This gives a Body Mass Index (BMI) equal to
Mrs. X3 is also very fearful. She is confused how to take the Fosamax; she has refused on
24. Standing upright with the back against the wall, Ms. X3 was able to have her back
and off to take it. There is a history of hot flushes. Her face turns red and also has trouble
and shoulders firmly on the wall. When asked to bend down and touch her toes, the
keeping her head upright. Recently, she also complains of positional vertigo, which she has
patient reached to the ankles (level 2), and reported no pain in any location.
had on and off since the past October.
Laboratory Findings:
Calcium Level is Normal; Protein Electrophoresis is Normal; Alkaline Phosphatase is Normal; and Thyroid Stimulating Hormone is Normal.
Physical Examination: There is not tenderness on the lateral rotation of the cervical spine.
There is mid-dorsal tenderness to percussion. No neurological deficit and the lumbar spine is
Impression:
normal, so is straight leg raising.
Ms. X3 has Severe Osteoporosis in the spine and Severe Osteopenia in the hip.
Impression: Obviously, this lady had not made any progress. She should be treated with
Fosamax. We will try to encourage her to do so and I will be getting back to you in the ne ar
She should have physical exam, dietary history, exercise assessment, fall assessment. She
should be very careful not to trip and fall, hence avoid fracture. It is important that Ms. X3 follow a calcium and vitamin D rich diet and exercise regularly. It is recommended that
this patient take Actonel, 35mg daily, and make another blood test, dexa after 0.5 years.
Best personal regards,
Dr. Z, MD, Osteoporosis Specialist
Knowledge Management & E-Learning, 6(4), 472–492
Table 2d
Specialist report for patient X4
Date: 6/25/2004
Patient: Patient X4
Date seen: 6/25/2004
Thank you for allowing us to assess your patient, Ms. X4.
Ms. X4, age 68 has a family history of Osteoporosis (Mother) and fractures (Mother, Hip).
I recently had the pleasure of examining X4. As you know, the patient is 65 years old, lives
History:
in Montreal, and was born in Greece.
Ms. X4 has no T-Score value registered before the last test. Based on the latest DEXA dated 1/11/2004 9:03:13 PM the Tscore of the spine was -1.8 and that of the hip was -
3.4. Ms. X4 is taking Calcium pills, 500mg, twice a day, Fosamax, 70mg once a week. There is no pain reported in any of the bones.
Past medical history is as follows: She suffers from osteoporosis and is treated with Fosamax and Calcium. The patient has been followed by me since 1994 for a multi-nodular goiter.
Ms. X4 did not suffer from any diseases in the past, and presently suffers from Arthritis or pain in the joints, and Hyperthyroidism. The patient is post-menopausal. Her menarche
There is no heat or cold intolerance, no change in weight, no change in hair loss, no visual
was at age 12 and menopause was at age 51.
problems, and no GI complications. The patient has no allergies. She is Hepatitis B positive.
Physical Examination:
Ms. X4 is 1.64m tall and weighs 54 kg. This gives a Body Mass Index (BMI) equal to
Physical Examination: Weight 121 lbs
20. Standing upright with the back against the wall, Ms. X was able to have her back and
shoulders firmly on the wall. When asked to bend down and touch her toes, the patient reached to her ankles, and reported no pain in any location.
Extraocular movements are normal. There is no exophthalmos. The thyroid gland was felt
and there is a large nodule on the left. There was no lymphadenopathy or splenomegaly. The
Laboratory Findings:
skin was smooth. Deep tendon reflexes were unremarkable. Cardiovascular exam was
Calcium Level is Normal; Protein Electrophoresis test was not done; Alkaline Phosphatase
is Normal; and Thyroid Stimulating Hormone is Normal.
Impression:
Laboratory Investigations: TSH 2,34
Microsomal antibodies 11
Ms. X4 has Advanced Osteoporosis in the spine and Osteopenia in the hip. BMD given below may show fractures and structural abnormalities, such as scoliosis and compression
fractures. Ms. X4 does not have any fractures or abnormalities, however she has signs of compression fracture of L4 (spine).
Impression: Multi-nodular goiter, osteoporosis
Recommendation:
It is recommended that you give your patient a comprehensive dietary history or refer her
Recommendation: Because she is euthyroid, no medications were given. She is to continue
to a dietitian. Also Ms. X4 needs advice on exercise and fall prevention which can be done
on Fosamax and Calcium.
by a physio-therapist. She should be very careful not to trip and fall, hence avoid fracture. It is important that Ms. X continue to follow a calcium and vitamin D rich diet and
exercise regularly. The best treatment for Ms. X is Forteo but this medication is not available today in Canada. An alternative would be an intravenous injection of Zometa.
Ms. X should make another blood test and dexa after 0.5 years.
Hoping this is of use to you, I remain yours truly,
Best Regards Dr. Z, MD, Osteoporosis Specialist
Specalist, MD, FRCP(C)
R. G. Saade et al. (2014)
Table 2e
Specialist report for patient X5
Date: 6/25/2004
Patient: Patient X5
Date seen: 6/25/2004
This 85 year old lady has severe osteoporosis.
In 1989, she fell and fractured her left hip which required pinning. The patient is originally
Thank you for allowing us to assess your patient Ms. X5.
from Laconia, has been living in Canada since 1967. Most of her life was spent doing village
Ms. X5, age 97 has a family history of Osteoporosis and has had bone fractures.
work. She has always been behind in calcium, she does not particularly like milk or dairy
products. As far as exercise is concerned, she has always been active. It is unknown if there
History:
is a strong history of osteoporosis.
Ms. X5 has no T-Score value registered before the last test. Based on the latest DEXA
dated 5/14/2004 4:05:06 PM the Tscore of the spine was -3.9 and that of the hip was -
Past medical history includes that of cataracts in both eyes. She has also been hypertensive
3.9. Ms. X5 is taking Calcium pills, 500mg, once a day, Fosamax, 10mg daily. There is
for a number of years, controlled with Vasotec 2,5 mg daily.
some pain reported in the bones.
Ms. X5 does not suffer from any disease in the past and presently suffers from Arthritis or
Physical Examination:
pain in the joints,
Reveals a pleasant lady in no distress
The patient is post-menopausal. Her menarche was at age 55 and menopause was at 10.
BP 160/56 Pulse 89
Physical Examination:
Examination of her axial skeletal exam shows some tenderness on lateral movements of the
Ms. X5 is 1.47m tall and weighs 40kg. This gives a Body Mass Index (BMI) equal to
cervical spine and tender over T12-L1 to palpation
19. Standing upright with the back against the wall, Ms. X5 was not able to have her back
Straight leg raising was painful bilaterally
and shoulders firmly on the wall. When asked to bend down and touch her toes, the
There is some pain over the pretibial areas to palpation as well
patient reached to the knees (level 1), and reported Moderate pain in the lower chest.
The patient underwent density test, the results were atrocious. She is more than 5 standard
Laboratory Findings:
deviations below peak bone mass in the lumbar spine and almost 4 standard deviations
Calcium Level is Normal; Protein Electrophoresis is Normal; Alkaline Phosphatase is
below peak bone mass over the left hip area.
Normal; and Thyroid Stimulating Hormone is Normal.
Her blood tests were basically unremarkable.
Impression:
Ms. X5 has Advanced Osteoporosis in the spine and Advanced Osteoporosis in the hip.
Impression:
Recommendation:
She should have physical exam, dietary history, exercise assessment, fall assessment. She
Obviously this lady had a previous hip fracture and she is a sitting duck for another one. I
should be very careful not to trip and fall, hence avoid fracture. It is important that Ms. X5
have advised her on the importance of avoiding falls and started her on cyclical didronel
follow a calcium and vitamin D rich diet and exercise regularly. It is recommended that
this patient take Actonel, 35mg daily, and make another blood test, dexa after 0.5 years.
Specialist, MD, FRCP(C)
Dr. Z, MD, Osteoporosis Specialist
Source: http://www.kmel-journal.org/ojs/index.php/online-publication/article/viewFile/400/230
Annotation and Extraction of Relations from Italian Medical Records Giuseppe Attardi, Vittoria Cozza, Daniele Sartiano Dipartimento di Informatica Università di Pisa Largo B. Pontecorvo, 3 I-56127 Pisa, Italy Abstract. We address the problem of extracting knowledge from large scale clinical records written in Italian by physicians. We perform recognition of rel-evant entities such as symptoms, diseases, treatments, measurements, drugs and so forth, and then we determine their semantic relations. We developed suitable training corpora in order to apply machine learning techniques to this task. We report on experiments performed on medical data provided in the context of a regional research project on technologies for health care.
Forschung · WissenschaftRecherche · Science Assistant Editor Rédacteur en chef Jürg Meyer, Basel Urs Belser, Genève Tuomas Waltimo, Basel Rudolf Gmür, ZürichPeter Hotz, Bern Advisory board / Gutachtergremium / Comité de lecture P. Baehni, Genève K.H. Jäger, Basel M. Perrier, Lausanne J.-P. Bernard, Genève